COMPOSITE (B-SEJ-242.UGR20)
Fact Sheet
Principal Investigator
Angeles Sánchez (UGR)
Research Team
- Eduardo Jiménez-Fernández (UGR)
- María José Ruiz-Martos (UGR)
- María Victoria Navarro-Hernández (UGR)
Work Team
- Antonella D’Agostino (Parthenope University of Naples, Italy)
- Laura Neri (University of Siena, Italy)
Fields of Science
Social, Economic and Legal Sciences (SEJ)
Duration
July 1, 2021 – June 30, 2023
Programme
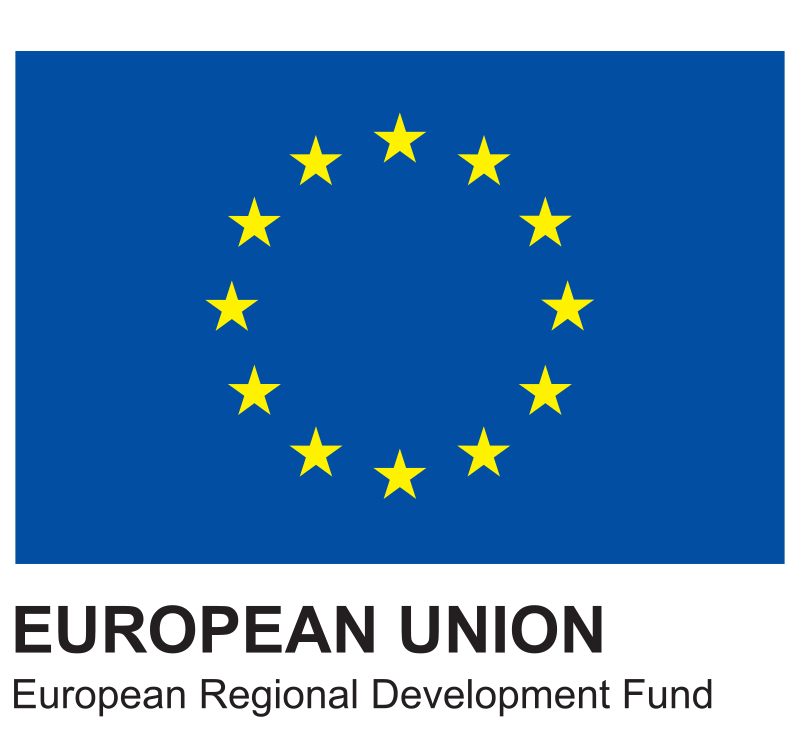
R&D&I Projects of the Operational Program ERDF 2020
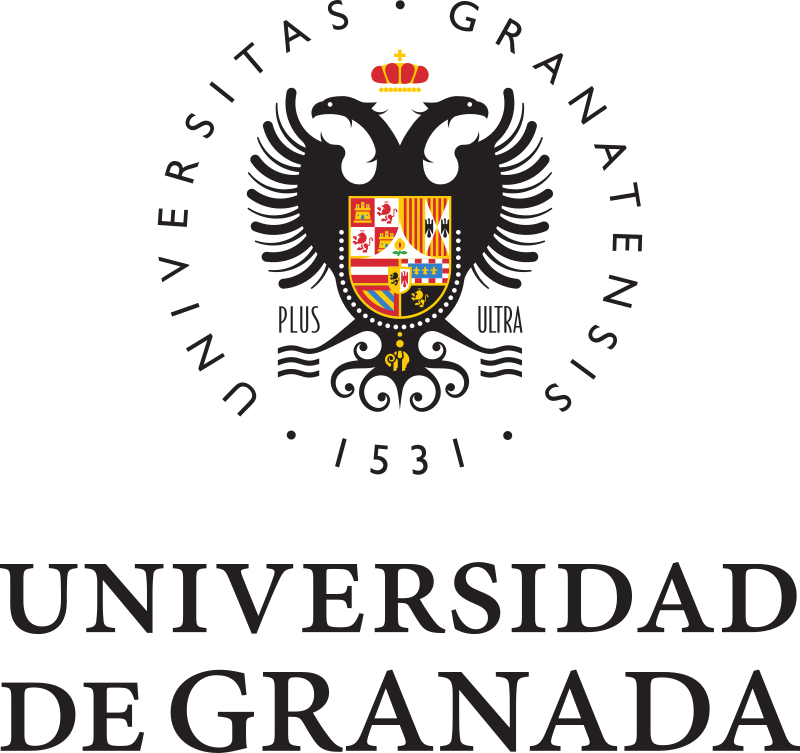
University of Granada - UGR (Spain)
Keywords
What is COMPOSITE about
Multidimensional Poverty
Child Poverty
Composite Indicators
Machine Learning
Simulation
Mathematical Properties
Funded Under
Challenges of the Andalusian society
Inclusive, innovative and reflective societies.
Health, demographic change and social welfare.
Objective
An Innovative Methodological Approach
The objective of this project is to develop an innovative methodological approach for the construction of robust composite indicators for the measurement, study and monitoring of poverty in Andalusia, both for the entire population and for children.
To do this, the methodology we propose is based on two basic pillars:
- The concept of metrics or distance.
- Machine learning.
01 – Metrics
Firstly, the metrics or distances (Euclidean or also fuzzymetrics) between the simple indicators and a target make it possible to carry out benchmarking. Despite their usefulness for monitoring and benchmarking across different units of analysis (groups of people, territories, etc.) and over time, most existing composite indicator methodologies lack a consistent mathematical structure to address this benchmarking challenge.
02 – Machine Learning
Secondly, the use of highly innovative mathematical and computational machine learning techniques will allow us to effectively identify patterns of redundancy in the information provided by the different indicators of poverty and deprivation. This is probably the aspect in which the most used methods to study poverty, such as head-counting, Distance P2 and data envelope analysis, provide unsound results. Likewise, simulation methods will be developed to test the robustness of the results.